Disambiguation by another feature
Assume that two features
and activate the neuron with opposite signs;[^1] and activate the neuron with the same sign, but some other feature can help disambiguate them.
Toy model
Model
The model has three layers (input, hidden, output):
input coordinates; hidden coordinates, with regularization on “activations”; output coordinates, with biases and ReLU.
The encoding (input -> hidden) and decoding (hidden -> output) weights can be tied together for simplicity, and this is what we’ll assume for the rest of the write-up, even though I think the story is slightly more natural without tying (because then each output can individually choose to use the disambiguation neuron or not).
Task
Input data
The input vectors
That is, we choose either the first half or the second half of the coordinates at random, and turn on exactly half of the coordinates within that half.
Output and loss
- Autoencoder task: the output is equal to the input.
- The loss is the squared norm of the difference between input and output.
Possible solution
Here is a description of possible trained model that gets zero loss despite having some pairs of features be represented on the same hidden neuron and with the same sign.
- One “disambiguation neuron” which has small positive weights on the first half and small negative weights on the second half (specifically,
and ).- This contributes
to the output of each feature that’s in the chosen half, and to the output of each feature in the other half.
- This contributes
- Other than that, each input feature is represented in exactly one hidden neuron, under the constraints:
- no two features from the same half share the same hidden neuron;
- each hidden neuron represents at most two features (from different halves);
- all weights are
.
- All biases are
.
Schematic view of the trained model:
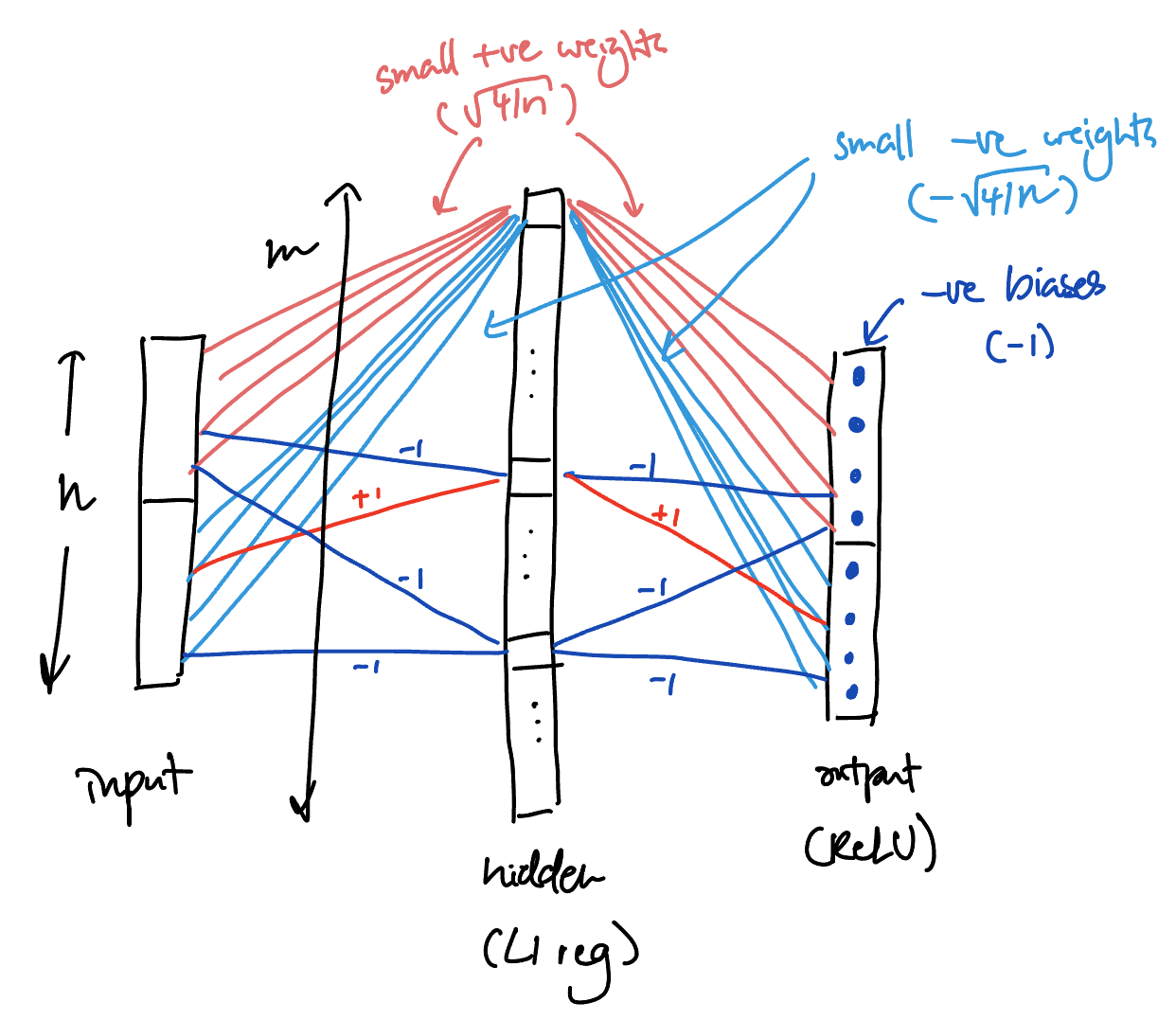
Schematic view of the weight matrix:
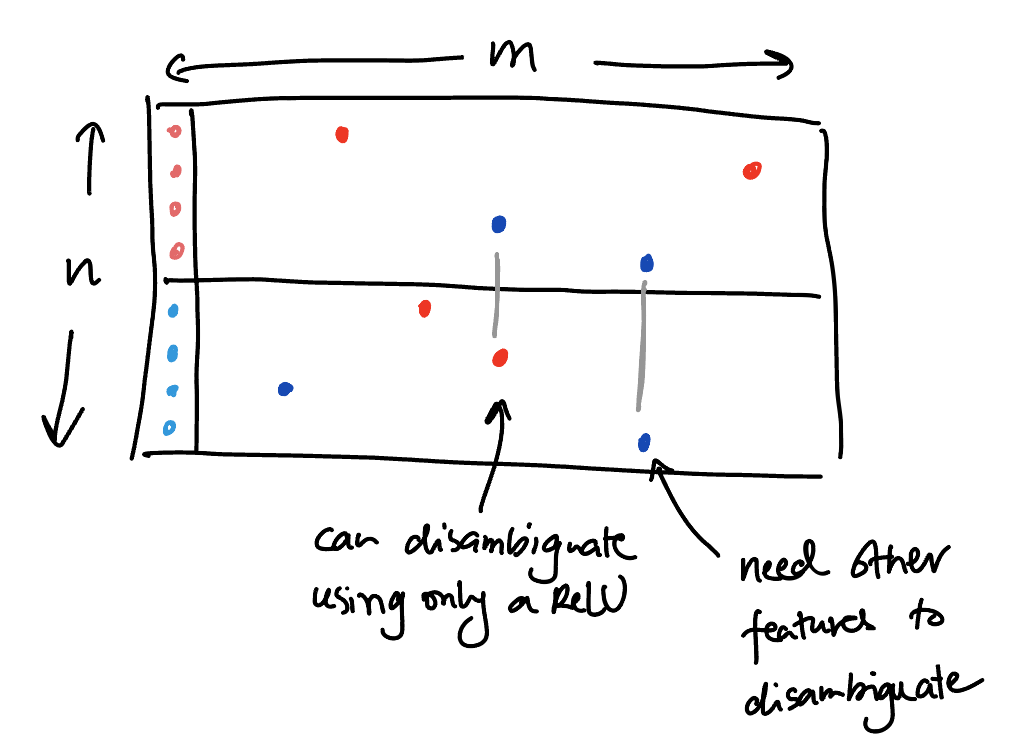
This works because:
- The output of all features in the chosen half will be
if the feature is on, if the feature is off.
- The (pre-ReLU) output of all features in the other half will be
if the hidden neuron that the feature is represented on gets turned on, otherwise.
Disclaimers:
- I am not claiming that this covers all possible zero-loss solutions (it clearly doesn’t: for example you could make the weights on the disambiguation neuron bigger and the bias correspondingly bigger).
- I am even less sure that SGD would find such a solution.
- I am not even sure that this solution is stable once taking the pressure from the
regularization into account. [^1]: note that this only really makes sense if the neuron does not have a non-linearity